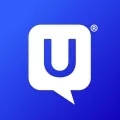
You have a user study coming up, but you don’t know which type of test to perform. Do you A/B test or use a multivariate test? Let’s take a closer look at each to find out more.
Before we start, let's define the key terms used in this article.
A/B testing compares two variations of a single element within a single design. The variants may be the color of a button, the label on a button, or the position of a button.
The key to the A/B definition is that the proposed differences are nuances to one—and only one—element within the entire design. But if we look at three or more variants, the test is still an A/B test, but now we call it an ‘A/B/n’ test, where n is the number of variants.
If we look at two or more aspects of a single element, such as button color and button label, or if we look at two elements, such as button variants and text content variants, then the test is considered to be multivariate, or MVT, rather than A/B/n.
For A/B testing, changes to one (and only one) aspect of a single element are done between variants. For example, the label on a button might change, or the button's color might change. However, if both label and color are tested in the same study, it becomes an MVT test. MVT tests can be very effective when looking at multiple design elements, although they can also become complex in both study design and analysis.
Both A/B and multivariate testing focus on behavioral rather than attitudinal metrics (though both can be collected). Neither testing type can review entirely different designs (such as two different website designs), only specific variants within elements.
Different overall designs can be tested using methodologies focusing on user attitudes, such as moderated or basic usability methods and tools.
For these big design explorations, use conversational UX methodologies, such as moderated testing, talk-out-loud usability (Basic TOL), or a mix of click-tests and survey questions to find out more about what the user likes and doesn’t like. Then, focus on the specific nuances through A/B or MVT testing.
It's also worth remembering what A/B and MVT won’t tell you:
A warning: it’s easy to throw out good design elements and retain poor elements based on limited and potentially erroneous data.
The image below represents an A/B/n example, where n=3.
The only change is the color of the comment bar element. More colors = larger ‘n’:
An A/B/n example
Now let's look at an MVT example, where n = 6.
Note that the only addition to the design element is the use of two label variants on the comment bar. Also notice that it doubled the n.
An MVT example with two label variants.
Adding a third element, such as a different label, no label, or a different typeface means the variances increase logarithmically—your ‘n’ explodes.
Consequently, the number of participants needed for a valid result gets much larger. A reasonable number of participants are needed for each variation, which means the effort of recruiting can quickly become staggering and may take a very long time to collect.
Our recommendation is to work incrementally on the nuances, building dependable and repeatable insights that can be used across the organization—and build on them with additional updates.
When you’re ready to test interactions, do that incrementally as well.
An A/B methodology can tell you which of two entirely different screen designs leads to better conversion rates. However, much is lost as you don’t know why one design is better than the other.
You may achieve more conversions in the short term but simultaneously reduce customer satisfaction by dropping beneficial features or elements that existed in the losing design (You may also have negative elements in a winning design that you don’t know about).
Use A/B testing when you want to know, statistically, how a nuance within your design affects user behavior.
You can add into the test attitudinal metrics around user preferences, likes and dislikes, and other opinions, while keeping your focus on measuring user behavior—that’s the magic of the hypothesis.
Conversion should be defined in your hypothesis. Design your test to focus on conversion, which could be buying a product, signing up for a newsletter, downloading a paper, registering for a conference, or any number of other commitments made by the user.
Of course, a simple click-through is rarely a conversion, but rather, a path to a potential conversion, which may be several steps later in the user process. Set up your test to measure both click-through and actual conversion.
The basic steps through an A/B-MVT test are straightforward.
Let's take a quick look at each stage of this process:
The A/B tests we talk about here are done as unmoderated tests that have automated criteria that include success validation. Automated UX testing can accelerate the pace of your UX research insights, increase the number of participants, and result in more confident design decisions.
We are focused on user behavior. It's important to define the automated success measures for the data either by URL or user action and should focus on your hypothesis—your metric—to show your hypothesis as true or false.
Assuming you’ve already set up your participant segments and screener, and you’ve set your task validation criteria, be sure to "test your test" with some of your team. Make updates and get stakeholder buy-in, then run the study for real.
When reporting the results, be bold, be clear, and support anything you say with your data.
Don’t be afraid to say that you don’t know. You can always do more testing.
You can also use some statistics to determine your confidence in your uplift—for example, you can be “90% confident in a 14% increase in conversion with an estimated error of +/—5%.” That means that you can be reasonably sure of a 9-to-19% improvement in your conversion rate with your new design.
It’s important to share what you learned from your study, even if it’s a ‘failure’—share with your team and your broader organization.
A report is not ‘happy speak’, it's truth, good or bad. Often user research is deductive: you build up a library of what doesn’t work, rather than what does.
Own your results, good or not, so that colleagues can learn from your great work and talk with you about it. That makes failure a success—and success even better.
Now let's take a look at a recent study we conducted. We used real participants but an imaginary brand that we created especially for this exercise.
In our example, let's imagine a company is looking at a program called TXT.
You'll also need to decide which variants are being tested
In this example, the "control" is the orange box, while green and gray are our variants: B and C.
Always state a control—this is likely to be the current design, already in-use.
This is the first test of this type for the product.
List any other known data here, both from internal testing and external resources.
For example, Nielsen Norman Group suggests that people are more likely to click on green (the green used here is a company brand color).
You may also need to factor in accessibility issues at this point - you can use free tools like Coblis to check for any color-blindness issues which may occur in advance.
Keep the statement very direct and measurable.
In this case, our hypothesis stated: "We believe a higher percentage of users will click on variant B (the green box)." If you include words like "and" or "because", your study metrics must prove each part of your hypothesis—the user actions and the motivations.
Metrics should align to the hypothesis. In this example:
Decide how you want to share your reporting. Is this for a select group of stakeholders, or should this be shared with the wider business?
You can choose how best to share results. For example:
This example should have given you a good understanding of the differences, advantages (and limitations) of A/B and multivariate tests, and enough information to begin creating your own, so what are you waiting for? Get out there and get testing!
Uncover human insights that make an impact. Book a meeting with our Sales team today to learn more.