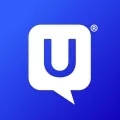
Demystifying Statistics: Why and how we adjust the p-value for number of tests run
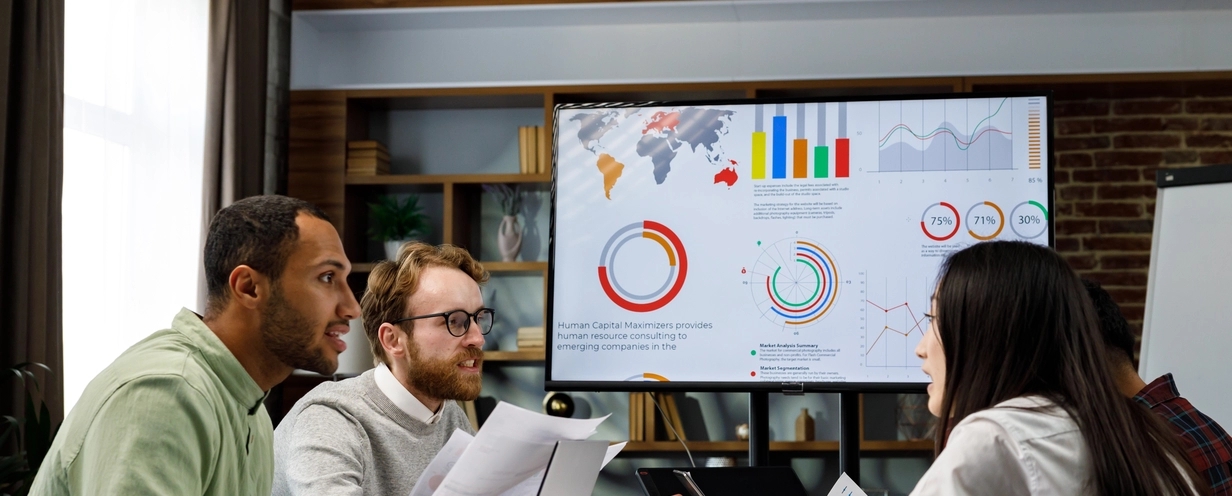
In the previous post in the Demystifying Statistics series we discussed what statistical significance means and what a p-value is. In this post we are following on with that discussion of p-values, and more specifically, we are investigating the number of statistical analyses we are running and how we should then adjust the p-value we use accordingly.
Recap on what p < 0.05 means
In the last blog post we discussed running statistical tests on the data you can collect in your UserTesting studies and how to interpret the findings.
If you have collected data which gives you averages that you want to compare, you can run a statistical test to see if there is a difference in the means. If you obtain a p-value of less than 0.05, we are happy to conclude there is a difference between our means, and they are statistically significantly different. If our statistical test came back with a p-value greater than 0.05 then we would conclude that there is no difference between our means, and they are not statistically significantly different.
Setting the p-value at 0.05, is the same as there being a 5% chance of obtaining the result from our statistical test, if there isn’t actually a difference there. If the p-value we obtain is less than 0.05, there is less than a 5% chance that the difference we have found isn’t really there. Our finding is unlikely to have happened by chance as it has a low probability of doing so.
The p-value doesn’t have to be 0.05 but typically in statistics it is the cut-off we are happy to live with. What we are setting with the p-value is how strict we want to be about saying there is a mean difference when there actually isn’t one there.
When we set the p-value at 0.05, this means each time we run a statistical test, we will only say there is a statistically significant difference when there actually isn’t one, one in every 20 times. Or in other words, 5% of the time when we run a statistical test, we will say there is a difference between our means when they actually isn’t one.
If we want to be more stringent to avoid making this mistake, we can reduce the p-value, for instance to 0.01. This would reduce the probability of saying there is a statistically significant difference when there actually isn’t to 1 in every 100 times when we run a statistical test.
So why do we need to adjust the p-value when running statistical tests?
As we just discussed, 5% of the time when we run a statistical test, we will get a p-value less than 0.05 and conclude there is a statistically significant difference, when there actually isn’t a difference in our population to find – we have just found it by chance.
This becomes a big issue if we are running a large number of statistical tests as our chances of making this mistake increases. So we know for each statistical test we run, we have a one in 20 chance of making this mistake.
Consider if we have multiple statistical tests to run. Imagine a scenario where we had our Design team draw up four different prototypes (A, B, C & D). They have asked us to compare the average ease of use ratings between each of the prototypes. To compare each of the prototypes with each other we would need to conduct six separate statistical tests.
When running multiple tests the probabilities accumulate, and for our six tests we end up with a 26.5% chance one of our comparisons would make this error; which is well above our usual 5% and is unacceptably high. We now have a one in four chance of making an error and saying there is a difference when there isn’t one.
How can you adjust the p-value?
What we can do this help reduce the chance of making an error when conducting lots of comparisons in our data is to correct the p-value. One correction we can apply to the p-value is to divide it by the number of tests we are planning to run. Really simple!
In our example, we would divide our usual p-value of 0.05 by six. This gives us a new p-value of 0.008, and when we run our statistical tests on our four prototypes, we would now only accept there is a statistically significant difference between any of our prototypes’ means if our tests obtained a p-value less than 0.008.
What we are doing dividing the p-value by the number of tests we want to run, is making it much stricter before we have enough confidence that the difference is truly there.
What does this mean for UX Research?
If you have a lot of data and want to make multiple comparisons, then adjusting the p-value is a very quick way to ensure you will be reporting back more robust findings to your team.
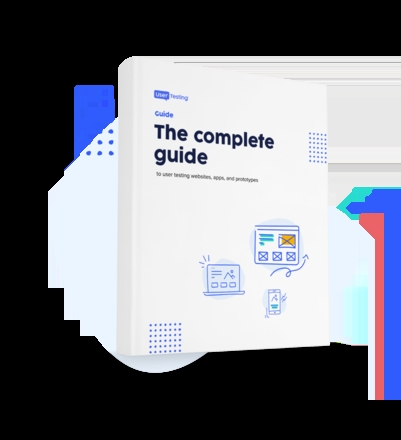
Get started with experience research
Everything you need to know to effectively plan, conduct, and analyze remote experience research.