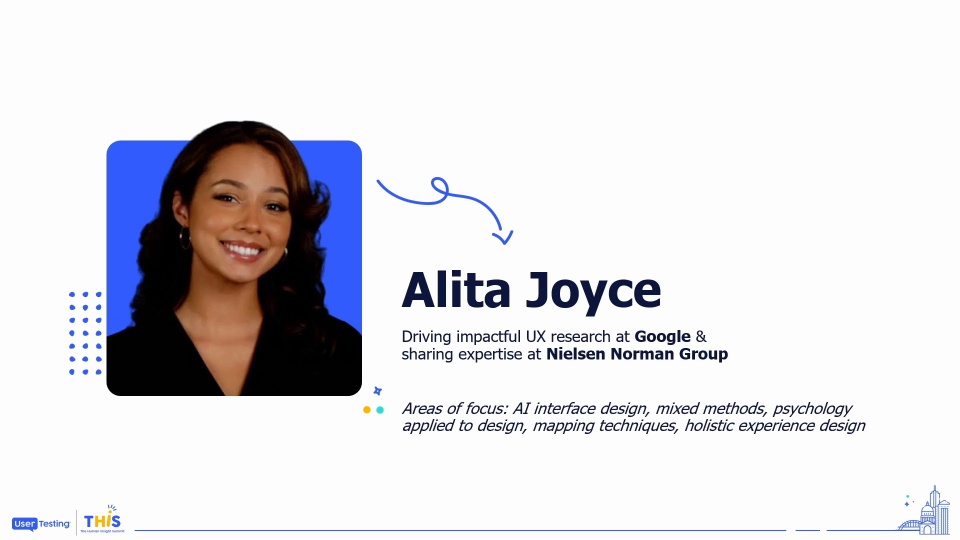
Alita Joyce
UX Researcher, Alphabet (Google)
Join Alita Joyce, UX Researcher at Alphabet (Google), as she shares real-world case studies and practical applications of AI in research. Learn how to leverage AI tools to streamline each phase of your research, from project planning and data collection to generating actionable insights. Attendees will receive a curated list of example prompts to kickstart their AI-powered research journey.
Thank you. Thank you.
Hey, y'all. Good afternoon.
One, a pleasure to be here. Super cool to see y'all, and thank y'all for being interested in AI. Today's conversation is going to be an incredibly practical overview of specific use cases for research.
I lead research for Gemini in Google Cloud, and so it's something that is amazing to work on as well as use and make me so efficient.
I'm wondering with y'all today, how many of you all are working in addition to sitting in in conference sessions today? Show of hands. How many are actively working as...
Thank you. Thank you.
Hey, y'all. Good afternoon.
One, a pleasure to be here. Super cool to see y'all, and thank y'all for being interested in AI. Today's conversation is going to be an incredibly practical overview of specific use cases for research.
I lead research for Gemini in Google Cloud, and so it's something that is amazing to work on as well as use and make me so efficient.
I'm wondering with y'all today, how many of you all are working in addition to sitting in in conference sessions today? Show of hands. How many are actively working as well? Right? Yeah. Ideally, you're not, but culture of do more with less type of thing. So let me tell y'all how I started my day.
Whenever I'm speaking, I like to have a long routine, get ready, take my time, listen to a podcast, some hype music, of course. This morning, I didn't get to do that. I got a fire drill, fire drill from my VP who, was like, yeah. We're thinking about this specific direction.
My PM, product manager, messages me and says, Alita, can you summarize all your insights related to this specific aspect of Gemini chat in Google Cloud Platform?
I'm like, well, yeah. Of course, I can. And what probably would have taken me if I had to say four hours before Gemini took me an hour. So those are the types of things that I wanna give you all today.
I have some very specific and practical use cases. And on that specific one, I have a insights document. It's my insights repository. It's probably a hundred pages.
It's not casual reading for my stakeholders, but it's a great knowledge hub for me. And so in that specific context, what I did, one, it's also very nice that this is public and I can actually say how I did this today, is Gemini and workspaces.
So I was able to open up Gemini in my Google Doc, my insights repository, and say, summarize all the insights related to this specific thing, create top five takeaways as well as some specific follow-up opportunities of research for me, which I will now be doing next week, and summarize that for me. So that using the insights that I created, Gemini summarizing that, again, reduced my time by at least fifty percent. So those are the types of use cases I want to talk with you all today. Again, thank you, Mike, for the introduction.
I am incredibly passionate about all things tech, design, experience, anything like that. My day job is, yes, at Google. I lead research for Gemini and Google Cloud Platform, accessibility, new market opportunities, and all that good stuff.
This past weekend, and I've worked with NNG for many years. Jacob continues to be one of my closest mentors. He taught me how to do UX, which is super cool to say.
And Nielsen Norman Group has such a special place in my heart, So you'll also hear some specific studies and cases from me throughout today's session on how that AI opportunity comes up there as well, particularly from my class this week where I had a really interesting attendee ask me. Basically, they have a two week sprint to get through something that should take four weeks. How do they do it? Spoiler alert. The answer is AI.
So in today's session, I'll give you a quick overview, set some baselines for not what is AI. I'm gonna assume y'all know what AI is given that you're here at this conference and in this room. But I'll do some baseline setting for us, and then we'll get into the fun stuff, which is going to be explicit prompts. I do have a bonus resource for you all that I crafted that is specific prompts that you can use.
Though I work at for Gemini, I want this to be applicable no matter the specific generative AI model that you're using. So whether it's Copilot, ChatGPT, OpenAI, Gemini, whatever it is, I want you to leave with specific prompts that you can use to get started with, particularly because we're in this culture of do more with less, less time, less people, less resources, obviously, less money, and also less energy. Like, is anyone else just tired? Like, we're grinding.
The backlogs of work that we have to do are massive.
And one thing that is core to me as a researcher is my perspective of adaptability and gratitude. So reframing some of these problems of less, less budget, less time, all of those things. How can we start to use artificial intelligence to support us in this work, to act as our assistant? I don't want you all to leave this room today feeling scared of artificial intelligence.
Are you scared of your interns taking your jobs? Probably not. Should you be scared of Gemini taking your jobs? Probably not.
But I do want you to leave with some practical advice on how we can start to use this and put this into practice. How can I make Gemini, GPT, Copilot, whatever it is, work for me and make me more efficient? So given the ever growing presence of artificial intelligence, it's not going anywhere. How can we use it to make us more efficient?
So some things to know, just baseline setting for generative AI. Obviously, I'm not gonna go through a full SWOT analysis. This could take hours, and consultants are paid tons and tons of money to do this for companies, so I'll spare you all that today. But I do wanna call out the specific strength that is going to be the foundation for today's session, which is increasing efficiency and accelerating your delivery of insights.
So I'm gonna walk through a couple of the very specific use cases for us in AI today across a given high level research process. We'll walk through a thought experiment that is going to be consistent through all of those pillars, and then we're going to get into some specific just me sharing my own experience and specific applications. So this is going to be the strengths that we're going to focus on, accelerating analysis, giving us lots of insights, making us more efficient. And there is also this app, aspect of artificial intelligence that is bringing some objectivity.
For those of us that are trained researchers, that scientific method is consistent. Right? We wanna document our hypothesis so that we're aware of our own biases. And so this type of objective review that artificial intelligence can do can make us more aware of those biases and things like our reporting. So we'll talk about that in a section today as well.
Weaknesses, of course, it lacks context. It doesn't know your product. It doesn't know your culture. It doesn't know what your leadership wants. It doesn't know what your engineers want. You have all of that context, which is again why don't be scared of us replacing you. How can we use it to our advantage?
Biggest opportunities for us here, enabling some new research, democratizing access, which is actually how we'll wrap up today's class. I'm a huge advocate of democratizing access to research. And so as we end today's class, I'll give you some practical advice on how I'm actively doing that with my stakeholders right here, right now. This is obviously an ever growing, ever changing field, and so having this perspective of adaptability and a little bit of grit and per perseverance is really important to your success in adopting and using generative AI in your workflows.
Threats.
Of course, oh, scary AI. Right? So when it comes to threats of using generative AI, there's risk with everything that we're using. So the core thing here is to be responsible, be transparent.
You all know your corporations and your organizations the best, so it's your due diligence and your responsibility to say, how can I use generative AI in the context of my work? Please don't go and say, Alita, this researcher at Google said I can just use generative AI for all these things. Do your due diligence, please.
So check out all of those legal, privacy, security, compliance aspects before adopting any of those practices. That's a very important disclaimer for today's class.
That is one disclaimer. The second disclaimer that I want to give you all before we get into the heart of our conversation around specific prompts and use cases is that fact that this should be to support your existing workflows.
Design and research is incredibly complex. These are nuanced spaces that we're working with, whether you are working in an enterprise environment or consumer facing products. And so in this specific context, I want us to be aware that this is a supporting aid for us, not something for us to be fearful of.
Getting through today's conversation, I'm gonna walk us through a thought experiment. As I said, I'll give you some cases from my work, with Nielsen Norman Group as well as my work with Google working in Gemini actively and just a user of these products. But I'm gonna give us a consistent thought experiment that is this company called Power People. So welcome to the team.
Y'all are leading research for this company called Power People, which is a Chicago based. I'm from Illinois, so we'll say Chicago based, kind of staffing platform. So this is b two b. Some of our core customers that we're working with are large enterprises all the way down to small startups and fast moving tech companies.
So in this context of work, I wanna give us some specific opportunities for how a company like Power People can start to utilize generative AI to support them in research and delivering faster insights resulting in a better user experience.
So with our company, Power People, there are some different phases that we can rely on artificial intelligence to support us. If we generalize our resource process into these four key steps, for today's conversation, I'm gonna talk about free updates. I'll give us a quick overview of each one, but your entire prompt cheat sheet that you have as a bonus resource, I'll give you the QR code later in today's session. But in that specific context, these cheat sheets can be super helpful to actively saying how can I experiment with AI today?
First off, we want to define our problem space. What are we specifically looking to understand in this area?
What are our research objectives? Which methodology is most salient? For me, this also includes a significant literature opportunity and resourceful as a researcher. And so relying on what's already there, classic not reinventing the wheel and all that good stuff, this is happening for me at this defined phase of work. So some additional use cases that we can have here are generating research questions and hypotheses, helping us create participant screener and all of that. But I'll make this point now and continue to make it throughout today's session.
The two most available use cases for us with generative AI can be content creation and ideation as well as refinement. For me personally, as a researcher, my use cases of refinement are significant in this define and planning phase. My use cases for more content creation is happening more at this analysis phase. So you'll notice this dimension as we get through.
But in general, content creation and refinement are kind of the two situations that we're talking with here. And I'll also communicate ways that I use, my own data as training sets for the specific artificial intelligence that I'm working with. So we'll get started with our define phase. Of course, planning is, like, actively planning the specific study that you're looking at.
This can be any sort of test materials that you need for your sessions, interview guides, and things like that. For the purposes of today's conversation, I'm excluding conducting, but this is actually a great use case for user testing on some of user testing's artificial intelligence tooling as well. But for today, we'll skip that one, and we'll wrap up with our conversation on analysis.
This is where the biggest heavy hitting time saver is for me. So in this capacity, using things like session transcripts as well as my own notes, marrying the two and training a dataset on that to say, okay. Create a full session playback for me with this specific formatting I want. Call out these specific quotes.
That can really speed up not just your analysis, but also your reporting because I'm the type of practitioner where, like I said, I I will create a hundred page doc, but that's my knowledge power. I'm not gonna send that to my VP or my senior director of engineering. They're going to get the exact summary. Right?
But when I have that completed, very comprehensive view that is everything that I know about that experience, now I can consolidate that into a much more readable format for my exec team, which they love. And then when questions come up as they did this morning, I can easily pull from my larger dataset, my larger repository with much more speed, ensuring that I can create the impact that I need for our customers and ensure that we're making data driven decisions.
So in starting off with our first area of defining, I do wanna give us some context because you can't just jump in. I mean, you could just jump in and ask Gemini or ask chat GBT to say, can you create a participant screener for me in this context? But you will be much more satisfied with the results if you do some of these tips to create and write more effective prompts. The first is very much providing context. So all of my sessions with a specific AI module begin with defining the specific role and objective.
Who are you and what do I want you to do? You're an expert UX researcher in the context of a human resource management platform. Here are your customers. Here's what you're looking to do.
That's the type of context setting that will give you better outputs in this context. So definitely starting there. I give you some examples of this throughout today's session, providing that context, specifying the desired output. This is very helpful because if you have any sort of formatting requirements or formatting preferences, this will will directly put it into that.
The amount of time that I've saved in using something like a generative AI model to support me in formatting, unprecedented.
I you know, we're in UX. We can be very particular about our headings, our bullet points, the way things are written. And to be able to take something like a set of bulleted point research objectives and say, okay. Now expand on those with, like, two to three sentences for each and map out specific opportunities alongside each one of those can save an hour. And we can see this a lot even in other opportunities like the suggested prompts and things like Gmail. Those types of opportunities can really speed up some of our content creation as well as help us better refine the content to meet certain expectations and formatting preferences.
Setting any sort of constraints, so limitations on time, length, anything like that. I'm just coming out of our annual review cycle at Google, and you better believe I use Gemini for this. I very vividly remember I live in Seattle, and I very vividly remember walking along South Lake Union and doing voice to text of, you know, here's all the things that I did this year and using Gemini to say, okay, now format that in three to five bullet points. Something that I really didn't wanna do.
I'm like, don't you all know my work well enough at this point? No one likes writing those type of performance review documents. But that was super helpful, particularly in a context of a constraint on length that you can have. No more than two hundred words, three hundred words.
That type of use case is also incredibly effective. It can just save us a lot of that mental time spent trying to analyze that. And then finally, requesting clear and concise language. Usually, I do this after the very first instance.
I like Gemini to be quite comprehensive in round one, and then I'll ask for that refinement after the fact as well as things like tone of voice. Because I have published so much work with Nielsen Norman Group, I actually use that as a training set for my specific tone of voice. A common critique of something like, any sort of generative AI model is you can kinda tell when it was written by generative AI. There's certain ways of formatting and communication that just feels like a bot wrote it.
And so training it on, this is how I speak, this is how I format my content, has also definitely increased the preference and the quality of outputs for myself. So if you have any sort of training data, things that you've published, past research reports that you're very pleased with, that's a really good opportunity to use that as training data as well.
Let's get into these use cases for very specific phases of research. So as I mentioned, this defined phase, very early on in our research life cycle, this can be an opportunity to generate research questions, identify or summarize relevant literature, develop project timelines, and things like that. These are actually all specific use cases that I've done in the past six months. Over the past seven and a half to eight weeks, I've conducted six rounds of research for Gemini and Google Cloud Platform as one person, which included a mix of qualitative studies and a survey as well as some additional activities.
There's no way, literally no way that I could have done that if I wasn't using Gemini for things like this, particularly because I'm sure some of you are like me and a bit of perfectionist. So if it has my name on it, I need the quality to be to be right. So when it comes to I don't wanna share my research report with, you know, senior engineers, senior engineering directors, and senior PMs without it looking a certain way. Gemini was incredibly helpful in that.
So as I mentioned, I'll use something like voice to text and just chart out here's exactly what I'm looking for and ask Gemini to clean it up, format it, improve those research objectives, use consistent formatting and bullet points, and things like that. Another really common use case for me has been in literature reviews. There are in highly mature research organizations, there will be so much research for you to comb through, and it's not realistic to read, you know, let's say, three hundred pages of work in order to directly inform the the types of research that you're looking to.
As I mentioned, I'm incredibly resourceful as a researcher, and I want to make sure that I'm targeting high value research opportunities.
Things like, any sort of generative AI model can be incredibly instrumental in in summarizing existing papers. So, again, you know, Gemini is now available in workspaces if you have that specific membership area. This was very common use case for me. So take these specific research decks, these specific research papers, consolidate them down into top five to ten takeaways, summarize any points related to, you know, insert topic that you care about in that time and moment, as well as identifying new research opportunities. Those are specific prompts that you can use in this context of defining your problem space.
If we think about our thought experiment then with power people as we're leading research for this HR area, let's say in this context we're looking to craft a participant screener. Now some organizations, depending on your overall research maturity, you may have standardized criteria and standardized screening questions at your disposal.
That's fantastic.
I still would use Gemini in this context and do some mapping. I think when people first get started with any sort of generative AI model, they come in with a bit of an all or nothing mentality. Either I'm gonna take it all or I'm gonna leave it all. I I don't want you to think like that.
It's not binary. You can ask these questions. The effort is low. See what you get back based on your specific experience and what you need to know.
Take it or leave it at, like, bullet point by bullet point. So this can be a really nice opportunity to say, you know, how exactly would I ask a question around trying to understand individuals who are HR professor HR professionals involved in hiring decisions? What is a closed ended question that I could craft in that situation? Again, if you have standardized questions, great.
But this is a really nice opportunity for us to think through that. This is another context to where for me as a practitioner, I use Gemini in these contexts or whatever large language model that you're using more so for content refinement and gap identification.
So I may put in my specific screener, say, here's my main objectives. What questions would you propose in addition to that? Again, sometimes they're really helpful of, like, yeah, that's a valid gap. Other times, it's like, no.
I don't need it. That's not relevant to me in my context. It's just adding bloat to the specific screening question. This is again where that critical thinking is so important.
It's not just cool. I'm gonna take it all. See what you can work with and use this as your intern.
I will say too, these are, specific prompts and outputs that you're seeing on all these screens. You get a copy of the slides. I used a mix of large language models because, again, I want you all to know you can do this in a lot of different contexts. But these are all actual outputs that you can receive in this context. So for this prompt of a participant screener, we see specifics on current job title. That's an easy one targeting those HR practitioners specifically.
In the context of planning our actual study, some use cases here are developing that structured plan, which can be incredibly helpful in context like interviews.
You can also do any sort of participant communications. I actually do this pretty frequently of, when I'm working with large scale customers rather than, doing a batch send of invites. I create more personalized messages based on my specific engagements with them, and Gemini has been incredibly instrumental in that situation as well. So any sort of participant communications, introductory content for a survey or a screener, those can all be great. Again, you may have these existing as standardized templates in your organization, but this can be another opportunity consistent formatting? Those are really good opportunities here just to give us more polished deliverables and create a better, comprehensible summary of information.
Any sort of study guides can also be really helpful if, say, we're looking at exploring in our HR context. We're looking at exploring a specific segment of the industry or a specific user segment. We can say, okay. Summarize, what are the key competitors in this space? The SWOT analysis and things like that, you can also do to say, get a baseline of some of those opportunities and do your due diligence and do the work as well and do that kind of comparison.
When it comes to planning in the context of our power people kind of HR tooling example, let's imagine that we want to do some interviews with this user segment, some HR decision makers. We're looking for a foundational understanding here. This is a great MVP type of question to use for generative AI for a foundational interview study with a user segment that is pretty well understood in industry. If you're working in something more niche, obviously, this use case is a little bit less helpful, truly like cutting edge things.
It becomes a little bit harder. But in context like this, this can be a really nice starting point for you all. Again, in crafting out some of your initial documentation here, this is another case where I would apply my own questions and ask your large language model. What gaps do you see here?
What questions would you propose? And, again, it's acting as your assistant, acting as a colleague. When everyone is so busy, backlogs of pings, backlogs of emails, there isn't always this time for us to review each other's work. Sometimes there are or your cultures really support that.
Fantastic. But that's not always the case. So this can be an additional layer of gut check that you're doing with yourself as a practitioner.
So in this context of asking for a detailed research plan, you can also use this to help you create templates for yourself. Those of you that have been practicing researchers for a while now, I hope you're at that phase of creating operations and efficiency perspectives for yourselves, any sort of note taking templates, research templates, study guide templates, literature review, research programs, anything like that. If you don't have those types of templates for yourselves as practitioners, these types of large language models can help help you create those and apply your own formatting to them.
I'm known in my team and throughout GCP of having a very specific style to my documents, which is very much informed by my time at GCP, or I should say at Nielsen Norman Group. But in GCP, people know, like, it's mine. My things look the same. The formatting is pretty constant.
The section titles are the same. If you see something called insights repository, it's probably me in that specific space. And so so having these templates and using Generative to help you create these templates can also enable you to create kind of a personal brand around those. That when people see them, they're like, okay.
That's Sally's. That's Mark's. Fantastic.
This is another example of an output that we can receive here in this context of crafting a research plan. In this situation, there are a few things that I want you to have top of mind.
One is, again, this comparison. So what information is missing here for you? What information is top of mind to your stakeholders? So this is where Gemini and these types of large language models are lacking your specific organization's context.
So definitely apply that when you're interpreting the outputs here. Have your starting point draft, put that into a large language model, ask it to refine, but coming in with some bullet points is really nice. I'm not saying have this be your starting point for everything. As I mentioned, for me as a practitioner at this define and planning stage, usually my specific workflow is typing out some notes in a meeting of what we're looking for, doing voice to text, doing basically a mind dump of, like, here's everything that's top of mind for me, and then having a large language model, refine it, and putting it into a clearer and better context for readability.
Analysis is my biggest use case here. So, specifically, how I've done this for the, what did I say, six phases of research that I've done in, like, seven and a half weeks. In that context, for every single session, I have a note taking document. My note taking document, I've used since the beginning of my career.
I'm pretty sure I got this from Jacob Nielsen, and it has it's a spreadsheet, and it has three columns. The first column is a time stamp. This is like a Google Sheet format. The first one is a time stamp.
The second column is a specific question that I'm asking, which honestly, half the time I use it, half the time I don't. And then the third column is the specific note taking area. Fourth column is any sort of tags. So I have mental notes of if I'm using quotations, this is a quote that I probably wanna pull in for any sort of reporting.
If I'm doing, exclamation marks, that's my flag to say super interesting. That's a top takeaway. Make sure you go back to the recording. Question marks, follow-up question I should ask in session.
So with that type of note taking template that has those time stamps, basically, I'm very present in the session. That is my core focus.
Then I have session transcripts for every single research session that I conduct.
Using something like Gemini, I give Gemini both of them. So participant one, you're gonna get a copy of my note taking document as well as a copy of that session transcript. I asked the large language model, I want you to create a session playback with this specific formatting. For every single question that the researcher, insert researcher name, Alita, asked, provide a summary of the participant's response.
If you see the quotation marks in the note taking doc, pull in those quotation marks using the specific formatting. I always give an example of the output that I want it to have. So I give my specific example of an actual insight exactly from that study so that the model understands. But then I'm basically training the dataset on that specific participant.
Now with large language models today, I'm still a skeptic. So instead of having, a large language model look through all of my sessions at one time, I create new sessions within the large language model for each participant to reduce the amount of bias and kind of cross communication of insights from one participant to another. I learned that the hard way. So learn from me, at least for the time being. There is obviously a huge amount of gut checking and reviewing that comes into this context.
But for specific analysis, that's my workflow. And so for each session, I have the model create that session playback for me. I do probably thirty minutes or so of reviewing that entire document, formatting, cross checking specific quotes, any insights, any things that are standing out as strange to me. In my large insights repository document, basically, I have a separate tab for every phase of research that I'm doing.
Every single session playback goes into that document. I export that document, put it back into a new session for Gemini, train the model on that specific, you know, phase one research, all this session transcripts, which have been a hundred percent validated by me as the researcher, so the insights are solid. And then I'll start to ask follow-up questions. You know, can you summarize all of the insights related to this specific topic?
Or there's this theme, can you summarize insights related to here? I've actually done this in the midst of sessions too. So if I'm conducting sessions over two weeks, I will do this at the halfway point and then say, based on these specific research questions from this research plan, what questions are still left unanswered or do I need more data on? And that has been incredibly efficient because as you're actively conducting sessions, say, like, fifteen, seventy five minute sessions, the cognitive overload is massive.
You are consuming so much information. It's easy to miss things. It's easy to overestimate how much knowledge you have about a specific question. So that has also been a massive help for me in this context of analysis, but that's usually happening at that midway point.
As researchers, I feel we usually end every research report with here's more opportunities for research. This is kind of speeding that up to say, okay. Here's the questions that we still have answered or here are nice follow-up steps. But this type of mid study analysis can really save you a lot of time and make sure that you're answering all of your questions to the best of your ability.
In our power people example, we can think about a time of a similar situation. So providing that session transcript, saying, okay. Create that specific playback summary of me, and here's a specific example. This was fully generated by AI. So these specific prompts that you're seeing on the screen, the output here is a actual example. And I went a step further, and the entire Power People context of work, the business profile, also generated by AI.
I have worked with companies in this context a few times, so I gave it specific objectives of, like, here's what the company is trying to do. Here's a specific interview script that you can use. Create a hypothetical interview. I've trained the model on that hypothetical interview, and this is the output that it gave me. Now because I gave you that specific example of training the model on a hypothetical interview, I wanna call out a question that I commonly receive in my work at Nielsen Norman Group, and it is, well, you know, could we just have AI generate our personas? Could we just have AI generate our user insights or tell us, like, what is the top research that we should know about and have it be hypothetical data?
No. Like, generative AI is not a replacement for actual customer research. Again, the nuance is so important. And particularly in the context of behavioral data, the models are nowhere near the capability to actually observe a session and apply the layers of insight that we have. So this is a really good opportunity for us to take a session that a human conducted and your specific insights and notes that you have and parallel that with a session transcript or a session summary that has been generated by artificial intelligence.
Those are your three specific context of research opportunities using generative AI that can again speed up your processing and accelerate your information analysis.
I want to wrap up our session naturally by talking about transparency and democratization because I find some practitioners that are new to artificial intelligence may be hesitant to communicate and to be transparent about its usage.
I'm the opposite, and I think that this transparency and encouraging collaboration go hand in hand. When we're talking about transparency, I'm definitely communicating to my team how I used Gemini to support my workflows, the session transcripts and things like that. Particularly in a culture of continuous improvement and continuous innovation, that has been incredibly well received. I mean, I am literally researching for the product, so hopefully, I'm a user of it as well. But this kind of transparency is very important in the context of your organization.
And instead democratize access to these generative AI models and increasing efficiency, not just for you as a researcher, but for you as your entire team.
Specifically, how I'm doing this right now is for the massive insights repository that I have with my stakeholders, I'm providing suggested prompts to my product managers and to my engineers so that they're not always pinging me to say, can you summarize all the quotes related to this topic? Or can you pull any insights related to this? Because the models are trained on my specific documents, I have a moderate to high level of trust that those types of summaries will be good enough. If my PMs or my engineers or other UXers have questions, obviously, I'm accessible, so they'll reach out to me anyway. But this can encourage collaboration, increase access to generative AI, and also support people in just understanding the overall research that we have.
This is that prompt cheat sheet that I mentioned to you all. You should have access to it elsewhere, but please, by all means.
I curated this specifically for you, one, because as an instructor with NNG, additional resources are key. You know, this is nice to story tell the experience with you all, but I want you to have dedicated prompts as you're getting started guide. This again walks through examples of those phases that we talked through and specific prompts that I've used as well as a lot of those content refinement ones. The content refinement is so nice.
Of course, you can play around with these models however you want. I absolutely used them with my friends earlier this year to plan a trip for Peru. That's all fun stuff and great, but use it to support you in your workflow and use it as that intern that we talked about. As I close out today's section, a few tips for success.
Basically, I really want you to leave with this understanding of not being scared of AI, identifying some tangible examples, insert that cheat sheet again, and really focus on opportunities for you to adopt, adapt, and apply generative AI to support you in creating better experiences for your customers.
That's it. Thank you all so much.
Wow. It's beautiful. That was the most popular.
So I think the rule the biggest thing we took away from today is put her on the main stage next year. I think that's the biggest takeaway. If you're willing, I know you're really busy, but we maybe can do one question. She's speaking again in about eleven minutes. So one right there from the crowd. We'll get one in, and then I'll do the announcements. Here we go.
Thank you.
Have you experienced any skepticism from stakeholders when you're reporting out and saying that AI was used throughout your process? And if so, how have you how have you dealt with that?
I'll acknowledge my bias that is I'm literally working on Gemini. And so that skepticism, is appropriate because we know. But I think at this stage, we should a hundred percent maintain our level of skepticism, and that's where the critical thinking and doing massive gut checking is fine. Even with the amount of review that I do for any sort of output model, it's still reducing my time to deliver by a lot.
And so that transparency, again, of communicating what exactly went into it, what was the role of generative AI, and the output is really important. But I would argue that in a lot of context, people are still putting in less work than that to deliver certain things. And so I feel comfortable with the amount that I'm doing, but that's definitely at the practitioner level to set up your own process and expectations for that review to ensure that you're delivering quality and something that at the end of the day you're proud of. Because, again, it's your name on it.
So if you're not proud of it and you don't trust it, you shouldn't be delivering it to your stakeholders.
Yeah.